The intersection of Artificial Intelligence and investment is no longer a futuristic fantasy; it's a rapidly evolving reality reshaping the financial landscape in profound ways. AI's capacity to process vast quantities of data, identify patterns undetectable by human analysts, and execute trades with unparalleled speed is revolutionizing everything from portfolio management to risk assessment and beyond. Understanding the implications of this transformation is critical for investors seeking to navigate the complexities of modern markets and secure their financial futures.
One of the most significant impacts of AI lies in its ability to enhance investment decision-making. Traditional investment strategies often rely on historical data, fundamental analysis, and human intuition. While these methods remain valuable, they are inherently limited by cognitive biases and the constraints of human processing capacity. AI algorithms, on the other hand, can analyze massive datasets encompassing market trends, economic indicators, news sentiment, and even social media activity, to identify subtle patterns and predict future price movements with greater accuracy. These algorithms can be trained to identify undervalued assets, anticipate market corrections, and optimize portfolio allocations based on individual risk profiles and investment goals. The result is more informed, data-driven investment decisions that have the potential to generate superior returns while mitigating risk.
The rise of algorithmic trading is a prime example of AI's transformative power. High-frequency trading (HFT) firms have long used sophisticated algorithms to execute trades at lightning speed, capitalizing on fleeting market inefficiencies. However, the application of AI in algorithmic trading goes far beyond mere speed. AI-powered algorithms can learn and adapt to changing market conditions in real-time, making them more resilient and effective than traditional rule-based systems. These algorithms can also be used to execute more complex trading strategies, such as arbitrage, trend following, and mean reversion, with greater precision and efficiency. The increased use of AI in algorithmic trading has led to greater market liquidity, reduced transaction costs, and more efficient price discovery.
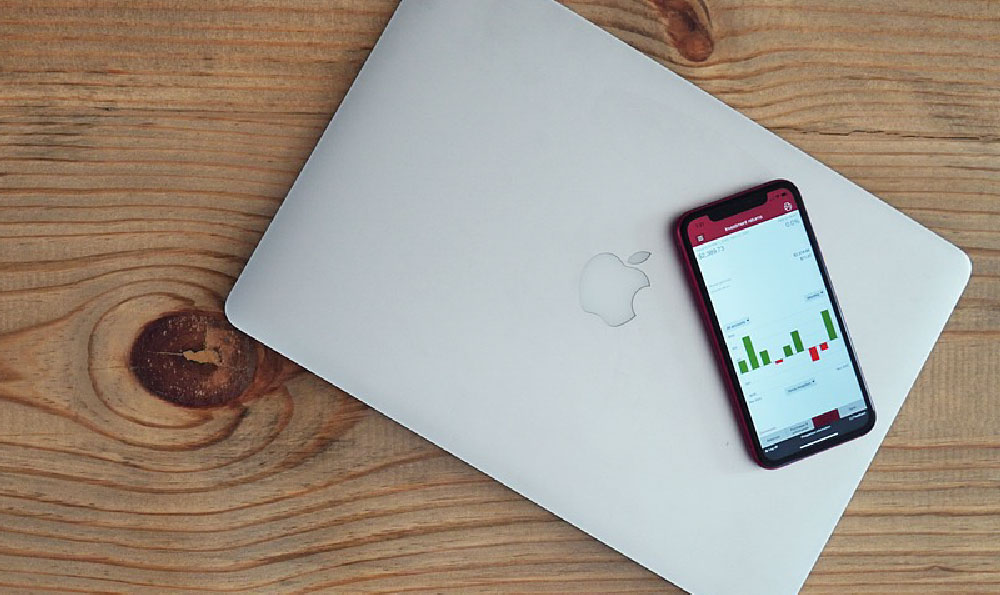
Beyond trading, AI is also transforming the field of portfolio management. Robo-advisors, which use AI algorithms to build and manage investment portfolios based on individual investor profiles, have become increasingly popular in recent years. These platforms offer a cost-effective and convenient way for investors to access professional investment management services, regardless of their level of wealth or experience. Robo-advisors can automatically rebalance portfolios, optimize asset allocation, and provide personalized investment advice based on individual goals and risk tolerance. The accessibility and affordability of robo-advisors have democratized investment, making it easier for individuals to participate in the financial markets and build wealth over time.
Furthermore, AI is playing a crucial role in risk management. Financial institutions are increasingly using AI algorithms to detect fraudulent transactions, monitor market risks, and assess creditworthiness. AI-powered risk management systems can analyze vast amounts of data to identify patterns of suspicious activity that might be missed by human analysts. These systems can also be used to stress-test portfolios under various market scenarios, helping investors to understand and manage their exposure to risk. By providing more accurate and timely risk assessments, AI is helping to create a more stable and resilient financial system.
However, the adoption of AI in investment also presents several challenges and considerations. One concern is the potential for algorithmic bias. AI algorithms are trained on historical data, which may reflect existing biases in the market. If these biases are not carefully addressed, they can be perpetuated and amplified by AI-powered systems, leading to unfair or discriminatory outcomes. It is therefore crucial to ensure that AI algorithms are trained on diverse and representative datasets, and that their outputs are carefully monitored for bias.
Another challenge is the lack of transparency and explainability of some AI algorithms. Many advanced AI models, such as deep neural networks, are essentially "black boxes," meaning that it is difficult to understand how they arrive at their decisions. This lack of transparency can make it difficult to trust and validate the outputs of these algorithms, particularly in high-stakes situations. As AI becomes more prevalent in investment, it is important to develop methods for making AI algorithms more transparent and explainable. This will require developing new techniques for visualizing and interpreting the inner workings of AI models, as well as establishing clear accountability frameworks for AI-driven investment decisions.
Looking ahead, the future of investment is likely to be increasingly shaped by AI. As AI technology continues to advance, we can expect to see even more sophisticated applications emerge, such as AI-powered financial planning, personalized investment recommendations, and autonomous investment management systems. These advancements have the potential to transform the financial industry and create new opportunities for investors to build wealth and achieve their financial goals. However, it is also important to be aware of the potential risks and challenges associated with AI, and to take steps to mitigate them. By embracing AI responsibly and ethically, we can harness its transformative power to create a more efficient, transparent, and equitable financial system for all. The key lies in understanding that AI is a tool, a powerful one, but a tool nonetheless. Its effectiveness depends entirely on the skill and judgment of those who wield it. Investors must therefore focus on developing their own understanding of AI's capabilities and limitations, and on using it to complement, rather than replace, their own knowledge and expertise. The future of investment is not about machines replacing humans, but about humans and machines working together to achieve better outcomes.